The Quiet Revolution in Observability
A quiet revolution has occurred in the ever-evolving landscape of technology monitoring and management. While industry giants like AWS, Google, and Splunk have long dominated the conversation around the traditional three pillars of observability – metrics, traces, and logs – a fourth pillar has emerged that’s fundamentally transforming how we understand and interact with our systems: Artificial Intelligence. At the forefront of this evolution, FusionReactor, a platform that initially carved out its niche in ColdFusion monitoring, has quietly evolved into something far more significant – a comprehensive observability powerhouse that’s not only embracing but advancing this fourth pillar, redefining what we consider the fundamental elements of modern system oversight.
The Traditional Foundation
Traditionally, observability has rested on three foundational pillars: metrics, traces, and logs. These components have been the backbone of system monitoring and analysis for years, providing the raw data needed to understand system behavior and performance. Metrics offer quantitative measurements that tell us what’s happening in our systems. Traces provide the breadcrumb trails that help us follow transactions across increasingly complex distributed architectures. Logs give us detailed records of events and activities that help us understand the narrative of our systems’ operations.
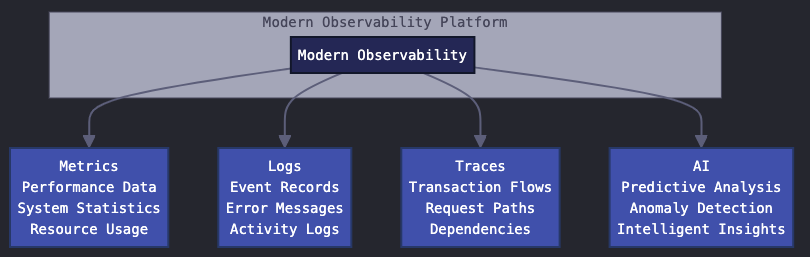
Emergence of the Fourth Pillar
However, the emergence of sophisticated artificial intelligence capabilities has introduced what we now recognize as a fourth fundamental pillar of observability. This isn’t merely an addition to the existing structure – it’s a transformative force that enhances and reimagines how the other pillars function and interact.
OpsPilot: AI in Action
FusionReactor’s OpsPilot exemplifies this evolution in observability, but what truly sets it apart is its ability to weave organizational knowledge into its AI capabilities. Rather than treating AI as a supplementary feature working only with real-time system data, OpsPilot creates a rich contextual understanding by incorporating an organization’s collective knowledge and historical experience.
This contextual intelligence is built through the integration of various organizational knowledge sources. OpsPilot actively learns from company documentation, such as README files, technical specifications, and architectural diagrams. It analyzes historical ticket data to understand common issues and their resolutions. Support questions, FAQs, and their answers become part of its knowledge base, allowing it to draw on past solutions when similar problems arise. Even team structures and responsibilities are incorporated, enabling the AI to provide insights that align with an organization’s specific operational practices.
Consider a scenario where an application experiences performance degradation. OpsPilot doesn’t just analyze the current metrics and logs – it correlates this information with previous similar incidents documented in ticket histories, consults relevant architectural documentation to understand potential impact areas, and leverages past resolution strategies that worked for similar issues. This creates a response that isn’t just technically accurate but is deeply relevant to the organization’s specific context and history.
This integration of organizational knowledge transforms OpsPilot from a generic AI assistant into a system that thinks and responds with the accumulated wisdom of the organization itself. When engineers interact with the system, they’re not just getting generic observability insights – they’re accessing an AI that understands their specific technology stack, knows their common issues, and speaks their organization’s technical language.
The Power of Integration
Introducing AI as the fourth pillar of observability isn’t just about adding new capabilities – it’s about fundamentally changing how we interact with and understand our systems. Through natural language processing, systems like OpsPilot enable engineers and operators to query their systems using everyday language, breaking down the barriers between human insight and machine data. Predictive analytics move us from reactive monitoring to proactive system management, while automated anomaly detection helps us identify issues before they impact users.
Beyond Simple Automation
The impact of this evolution extends far beyond simple automation or efficiency gains. By establishing AI as a fundamental pillar of observability, platforms like FusionReactor are creating new possibilities for system understanding and management. Machine learning algorithms can identify patterns and relationships that would be impossible for humans to detect manually. Predictive analytics can forecast potential issues before they manifest, while automated root cause analysis can dramatically reduce the time needed to resolve problems when they do occur.
A Journey of Transformation
FusionReactor’s journey from ColdFusion specialist to a comprehensive observability platform illustrates the broader evolution of the field. Through its OpenTelemetry integration, the platform can collect and analyze data from modern technology stack, from traditional CF and Java applications to cutting-edge cloud-native architectures. This broad coverage, combined with AI-powered analysis, creates a system greater than the sum of its parts.
The Future of Observability
Looking to the future, the importance of AI as the fourth pillar of observability will only grow. As systems become more complex and distributed, the ability to automatically analyze and derive insights from vast amounts of data will become increasingly crucial. The integration of AI capabilities will continue to deepen, with more sophisticated predictive capabilities and more natural ways of interacting with observability data.
A Fundamental Shift
This evolution represents more than just technological progress – it’s a fundamental shift in how we think about system observability. The addition of AI as a fourth pillar acknowledges that modern systems have grown too complex for traditional approaches alone. By embracing this change, platforms like FusionReactor are leading the way toward a future where observability isn’t just about collecting data but about truly understanding our systems in all their complexity.
The Path Forward
As organizations grapple with increasing system complexity and scale, this four-pillar approach to observability will become increasingly vital. The question isn’t whether AI will become a fundamental part of observability – it’s how quickly organizations will adapt to this new reality and embrace the transformative potential of AI-enhanced observability.
Conclusion
In this context, FusionReactor’s story is an example and a prophecy. It shows us not just what’s possible today. Still, it points the way toward a future where AI isn’t just a feature of observability platforms but a fundamental pillar upon which modern system understanding is built. As we move further into this AI-enhanced future, the platforms that embrace this four-pillar approach will be best positioned to help organizations navigate the growing complexity of modern technology landscapes.